Technological Revolutions & Artificial Intelligence
The transformative introduction and acceleration of generative AI technologies have made it abundantly clear we are on the cusp and in the initial stages of a paradigm-shifting technological revolution. In an increasingly complex, competitive, and dynamic environment, organizations must develop a firm grasp on the evolving technology landscape and how to best capitalize on existing and emerging trends.
What are Technological Revolutions?
Economist Carlota Perez has developed a framework for understanding the dynamics of technological innovation and its impact on society and the economy. With numerous historical examples, Perez’s framework offers a means of understanding and mapping technological revolutions and their underlying drivers. Applying the framework to the current technological environment, we can see that we’re just in the early innings of an AI-driven revolution that will drastically alter the business and technology landscape.
Lifecycles of Technological Revolutions
Perez’s framework outlines distinct phases across the lifecycle of technological revolutions. Each revolution begins with a set of interconnecting technologies that form a platform for future innovation. When a fundamentally disruptive innovation emerges within this cluster of technologies, it triggers a technological revolution.
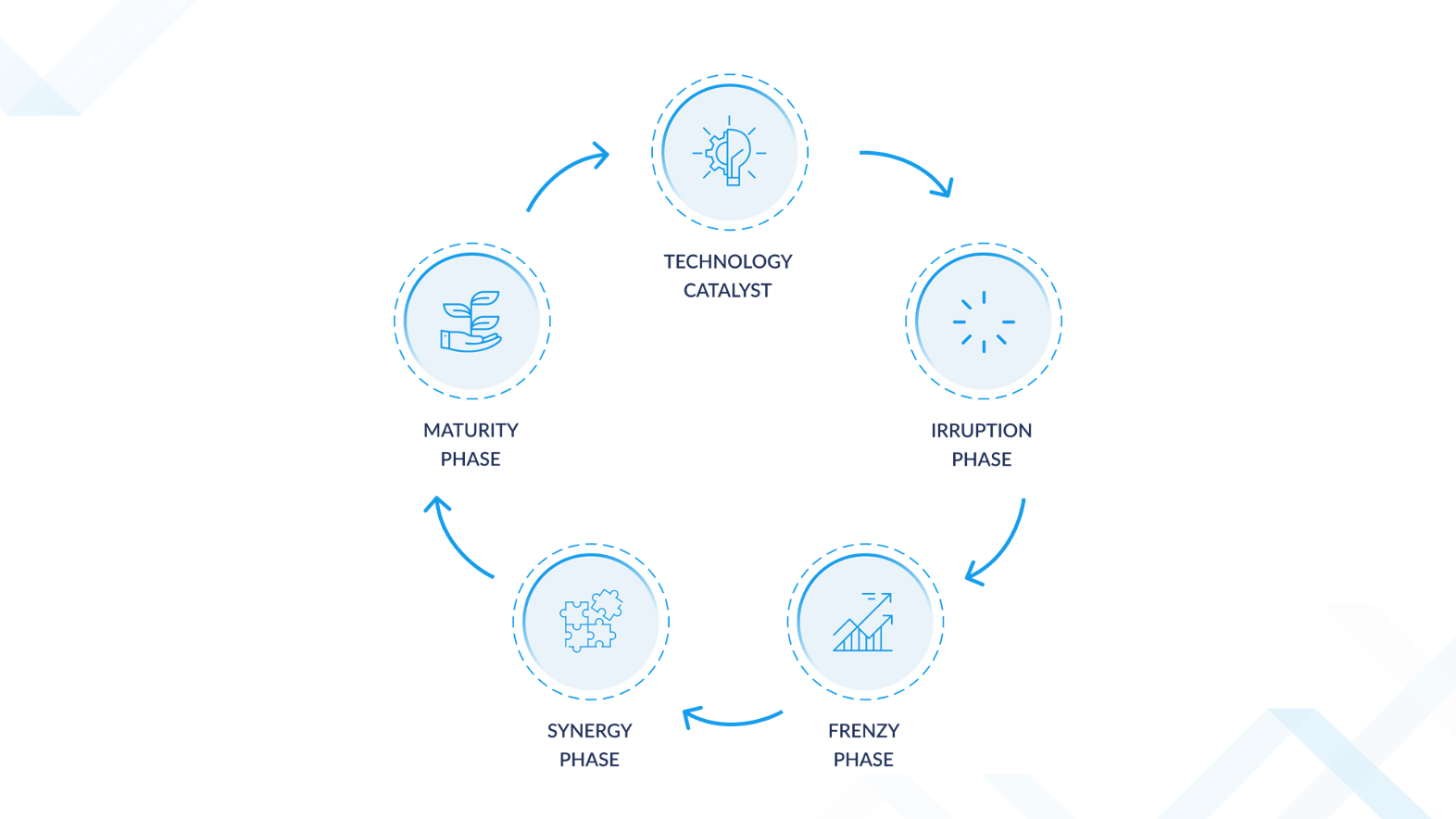
This trigger then leads to the following phases that form and underpin the revolution lifecycles, the first two of which represent the installation period and the latter two of which represent the deployment period:
- Irruption Phase: During this phase, new technologies undergo exponential growth and acceleration, with entrepreneurs and investors rushing to capitalize on emerging opportunities. This phase is also characterized by increasingly speculative investment activity, which can generate financial bubbles as optimism about the technology's potential drastically rises.
- Frenzy Phase: Frenzied financial growth accelerates catalytic technology's advancement and proliferation. Bolstered by an influx of capital, support, and resources, the rapid emergence of new systems, industries, and infrastructure generates increasingly momentous growth that is neither stable nor sustainable. Marking a turning point from the installation to the deployment period, the frenzy phase leads to some sort of financial decompression, market correction, or economic or geopolitical event, often accompanied by growing social and regulatory pressures.
- Synergy Phase: Following the inevitable corrective event capping off the frenzy phase is the synergy phase, characterized by more stable growth as the public, institutions, and markets adapt to a new technological paradigm. Often aided by policy, regulatory, and social changes, surviving and emerging companies introduce new successive generations of products, modernize existing technologies, and expand innovation. However, the synergy phase doesn’t always lead to favorable outcomes, as seen with WWII or the modern Chinese Communist Party.
- Maturity Phase: In the maturity phase, the technological revolution has primarily become a fully integrated and interwoven aspect of society and the economy. The innovative potential of the underpinning technologies has become realized through market saturation and widespread adoption. With relatively stable and sustained economic growth and technological advancement, this phase continues until the next disruptive set of innovative and transformative technologies that spark the irruption phase.
We’ve seen this lifecycle play out at least four times over the past two and a half centuries and very likely find ourselves on the cusp of a fifth. Even at a more micro level, we can see mini-cycles, such as with cryptocurrency or even virtualization technology. Although there are regional nuances, overlaps, and contextual considerations, the following table provides a high-level and generalized look back at some of the prior global technological revolutions:
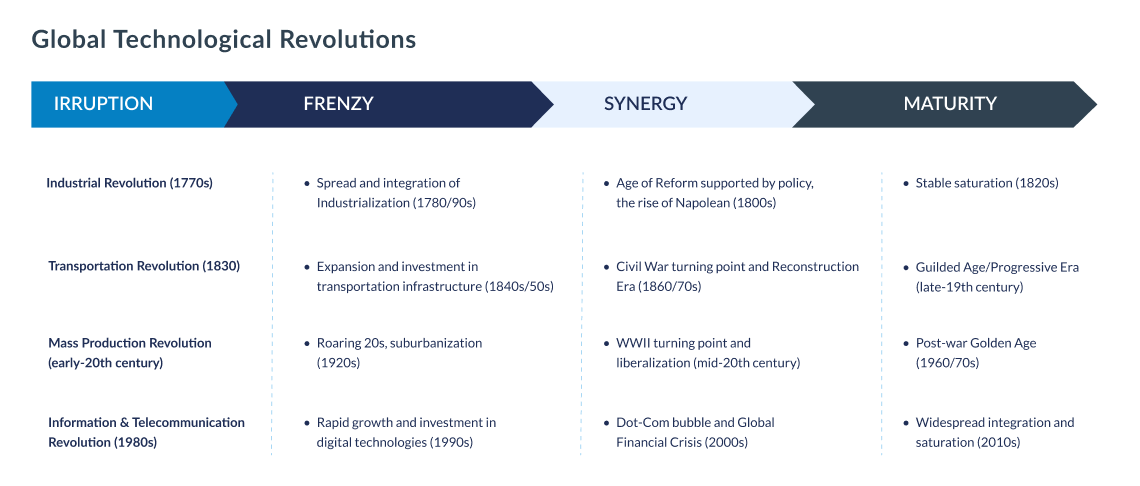
Installment Period
Several fundamental dynamics come into play during the installment period of a technological revolution, which follows the initial technological trigger.
First, critical technological resources become increasingly cheap and cost-effective. This cost reduction trend is historically associated with the rise and rapid development of innovative technologies (CPU costs leading to personal computers, storage costs leading to web and cloud services, etc.). As costs plummet, new technological innovation and advancement opportunities emerge, creating fertile ground for further progress. Just looking at one aspect of the current technology revolution, the rise and rapid advancement of AI and LLMs have the potential to lead to a drastic and dramatic collapse in the cost of software production.
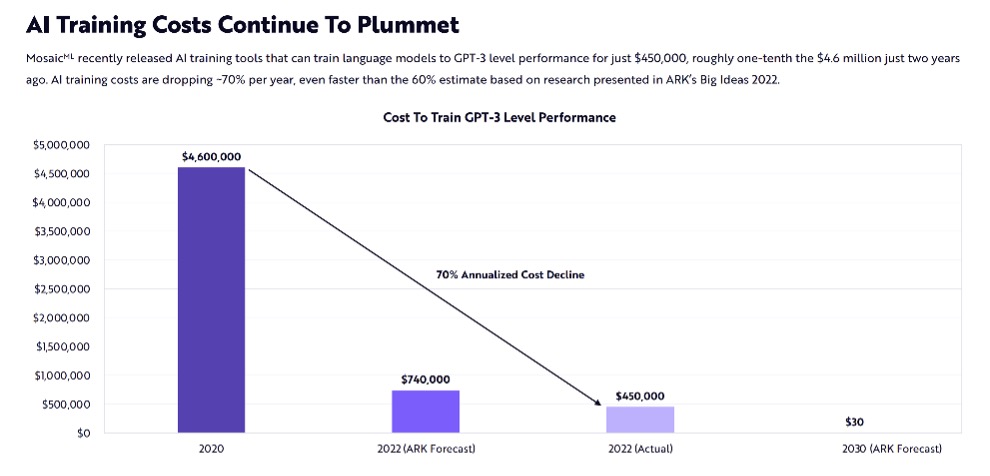
Second, two significant effects occur as these emerging technologies demonstrate their commercial and business value. As established incumbent companies begin slowly adopting these new technologies to protect and solidify their market positions, entrepreneurs and new entrants rapidly capitalize on the potential of these innovations, driving a surge in financial capital investment, particularly through venture capital and return-oriented investors. This influx of capital is instrumental in fueling the growth and development of the emerging technological paradigm.
Deployment Period
In the deployment period of the technological revolution lifecycle, consolidation and widespread integration of the new technological paradigm take hold. This phase represents a maturation and stabilization of the innovations.
Emerging technologies synergize and integrate with existing systems and institutions during this period. As frenzy-fueled financial capital pulls back, production capital fills the gap, focusing more on improving efficiency, production, and innovation rather than generating risk- and speculative-based returns. This capital infusion transition subsequently generates more sustainable, predictable, and healthy innovation.
At this point, government policies, regulations, and social norms are generally starting to take a more pronounced role in facilitating and enabling a more stable and aligned environment conducive to sustained innovation and societal integration.
The critical distinction in the deployment period is the shift from frenzied uncertainty and speculative growth to saturated integration and sustainable innovation. Established businesses adopt these technologies to enhance their operations, while entrepreneurs and new market entrants continue to explore opportunities within the existing paradigm. It's a phase of optimization and consolidation where the technological revolution's full potential is realized.
An Enterprise Imperative
Based on Perez’s framework and technological revolution lifecycle, we find ourselves in the early innings of an AI-driven technological revolution already having significant impacts across industries. AI technologies have spread like wildfire, fueled by an unrelenting flow of private capital, lofty valuations, and accelerating advancement. There is growing support from incumbents and startup entrepreneurs seeking to capitalize on the latest technology wave through emerging innovations, corporate investment, and internal system integration and development.
Based on the environment and revolution lifecycle positioning, as well as rapidly accelerating advancement, it is more important than ever for enterprises to develop robust strategies, practices, and technologies focused on the AI revolution. Doing so will ensure that they survive and thrive in the emerging paradigm.
Building an Enterprise AI Strategy
An effective enterprise AI strategy consists of two foundational cornerstones: the technology itself and the business practices that support and enable the use and advancement of that technology. By employing the following technologies and strategies, enterprises can position themselves to effectively survive and thrive through the paradigm-shifting technology revolution taking hold and unfolding.
The following strategy framework contains key technology and business practice focus areas for enterprises seeking to develop and deploy effective AI capabilities.
The AI Tech Stack
Although white-label services and solutions exist, many enterprises are exploring developing their own AI technologies. With this approach, they can build entire AI tech stacks specifically designed and tailored to their unique business needs, circumstances, and data. The growing momentum behind open-source technologies and solutions has served to reinforce the feasibility of this particular approach.
To build an aligned AI solution, it’s critical to first understand the essential components and infrastructure underpinning AI technologies. Looking specifically at generative AI, the technology stack can broadly be broken up into the following layers:
- Hardware Layer: This foundational layer comprises specialized computer hardware, such as graphic processing units (GPUs), Tensor Processing Units (TPUs), and Data Processing Units (DPUs), which are designed to handle the demanding computational needs of AI workloads.
- Cloud Layer: The other foundational layer is the cloud layer, which enables enterprises to operate AI-driven workloads effectively and efficiently. The cloud layer facilitates enhanced development, deployment, and operation by combining scalable infrastructure and service offerings, such as processing, storage, and networking.
- Model Layer: Trained on extensive amounts of data, statistical pattern recognition, and contextual understanding, the model layer is a core component of the generative AI technology stack. Models represent the engine behind AI applications, generating content and fulfilling functions based on model design and training data.
- MLOps Layer: Beyond the model layer, MLOps encompasses the set of technologies for optimizing, training, and refining AI models. MLOps solutions improve the model development lifecycle, enabling users to further tailor and train their models based on their specific and unique use cases and objectives.
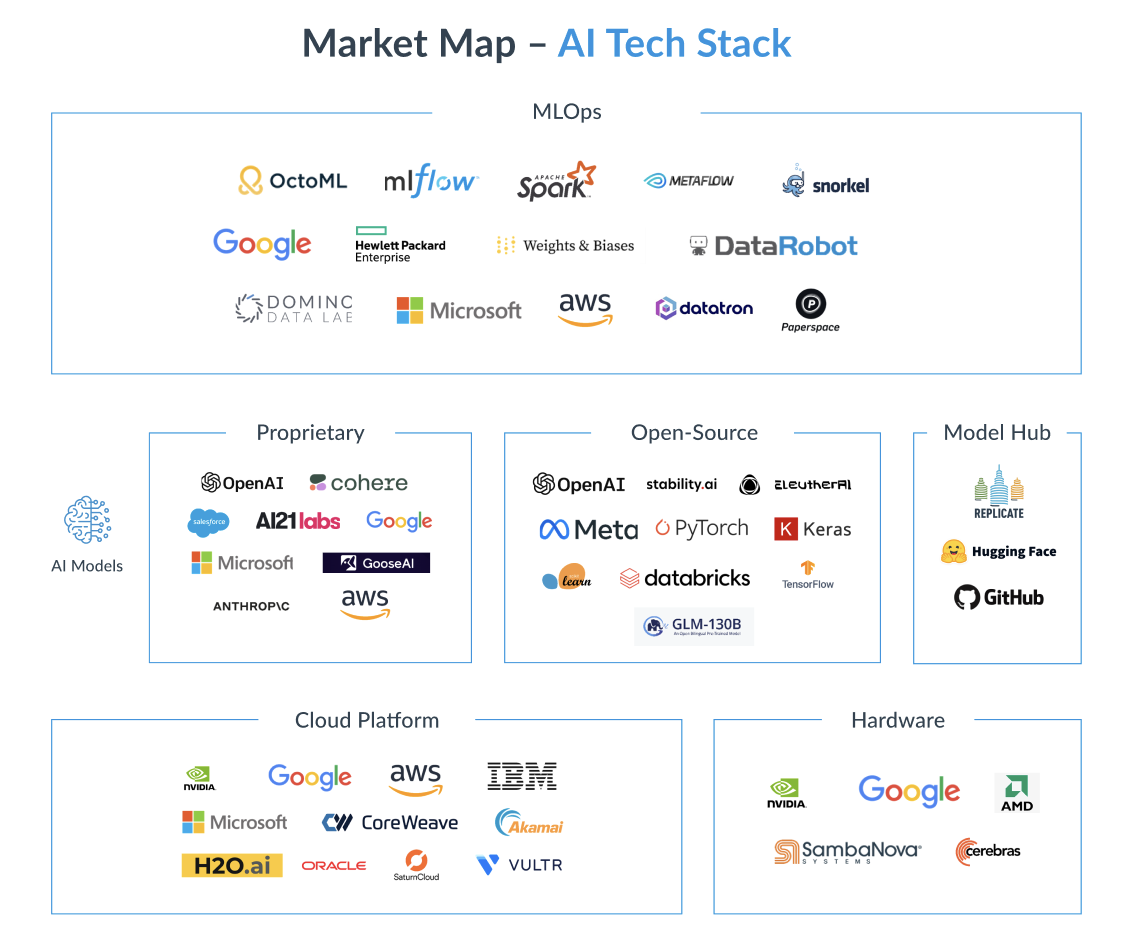
With a growing variety of solutions available at each layer, it’s important to ensure that selected technologies are aligned with business objectives and technological, infrastructural, and operational capabilities. It’s also important to consider integration needs and longer-term flexibility, adaptability, and functionality needs.
Responsible AI Management
Responsible AI is a comprehensive technology approach encompassing the tools, processes, and frameworks designed to ensure AI solutions' safe, ethical, and compliant use. Its primary goal is to mitigate potential misuse, abuse, and risks associated with AI while upholding human values, business ethics, and regulatory compliance.
In a period of increasing AI technologies, responsible AI plays a pivotal role in fostering public trust, enhancing security, and reducing legal and regulatory liabilities. By adhering to fundamental principles, including fairness, transparency, and accountability, responsible AI empowers businesses to make unbiased, well-informed decisions, fostering innovation and effective risk management. It enables organizations to gain a competitive edge and enhance operational effectiveness by improving their AI solutions' overall performance and reliability. Additionally, responsible AI is a crucial guardian of internal and external trust, guarding against the misuse of AI technologies that could result in legal and reputational consequences.
The following three key areas underpin responsible AI:
- Security: This facet focuses on safeguarding AI solutions through tools, processes, and frameworks that address security testing, jailbreak protection, compliance alignment, and threat visibility. It ensures the protection of AI systems during development, application, and deployment.
- Privacy: Responsible AI privacy technology encompasses tools, protocols, and measures dedicated to safeguarding sensitive data and ensuring compliance with privacy regulations throughout the AI lifecycle. This includes data protection, anonymization, and access controls, which build customer trust, preserve data integrity, and safeguard private information.
- Governance: Governance solutions for responsible AI enforce policies, guidelines, frameworks, and mechanisms to ensure the ethical, fair, and transparent use of AI. These tools often incorporate explainability features, bias detection and fairness mechanisms, and impact assessments to foster the development of AI solutions characterized by informed, aligned, and impartial outcomes.
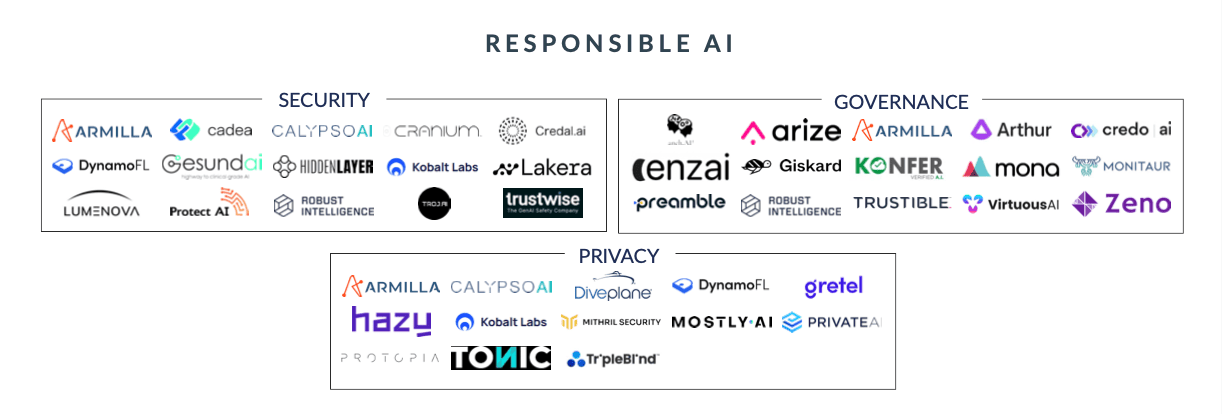
Enterprises will increasingly need robust AI security, privacy, and governance mechanisms to derive maximum value from their assets and achieve regulatory compliance, industry alignment, and consumer trust. Any piece of AI technology must be accompanied by technological guardrails that ensure that the technology accounts for each business's unique needs, objectives, risks, and considerations.
Enterprise Applications
With AI infrastructure in place, supported by security, privacy, and governance mechanisms, enterprises can now effectively deploy AI-powered applications tailored to specific business functions based on particular needs and circumstances. AIOps and conversational AI represent two applications that have demonstrated strong momentum in innovation and enterprise traction, particularly given their ability to generate greater resource efficiency, improved operational optimization, and effective data-driven decision-making.
Enterprise Application - AIOps
AIOps embodies a set of cutting-edge technologies and processes that leverage AI and machine learning to automate, optimize, and manage traditional IT operations and services. These solutions encompass diverse functions, including streamlined data collection, system monitoring, root cause analysis, service management, and suggested remediation strategies. The overarching goal is to enhance technological visibility and operational efficiency within enterprises.
Enterprises can accrue a multitude of benefits and competitive advantages through the employment of AIOps technologies. By automating and optimizing various IT operations, enterprises can unlock significant gains in operational efficiency, talent capacity, and resource utilization while reducing complexity. AIOps tools also serve a key function in automating the ingestion and processing of vast volumes of data from different sources. In doing so, they streamline observability efforts through reduced alert fatigue and intelligent incident prioritization. Often providing automated incident detection and rapid remediation, enterprises can reduce their downtime, disruptions, and cybersecurity risks.
Importantly, AIOps also empowers enterprises to scale the management of IT systems and resources efficiently, leading to improved service delivery and data-driven insights. Whether for ticketing and service desk interactions or asset discovery and change mapping, AIOps can alleviate the budgetary, talent, and resource strains many enterprises face.
These technologies drive efficiency, innovation, and competitiveness across IT operations. As connectivity, device ecosystems, and edge capabilities expand alongside a growing attack surface, AIOps will rise in importance, advancement, and adoption. Driven by accelerating AI sophistication, enterprises that employ effective AIOps solutions will be well-equipped to address the data, security, and IT challenges as they navigate the growing complexity accompanying the AI technological revolution.
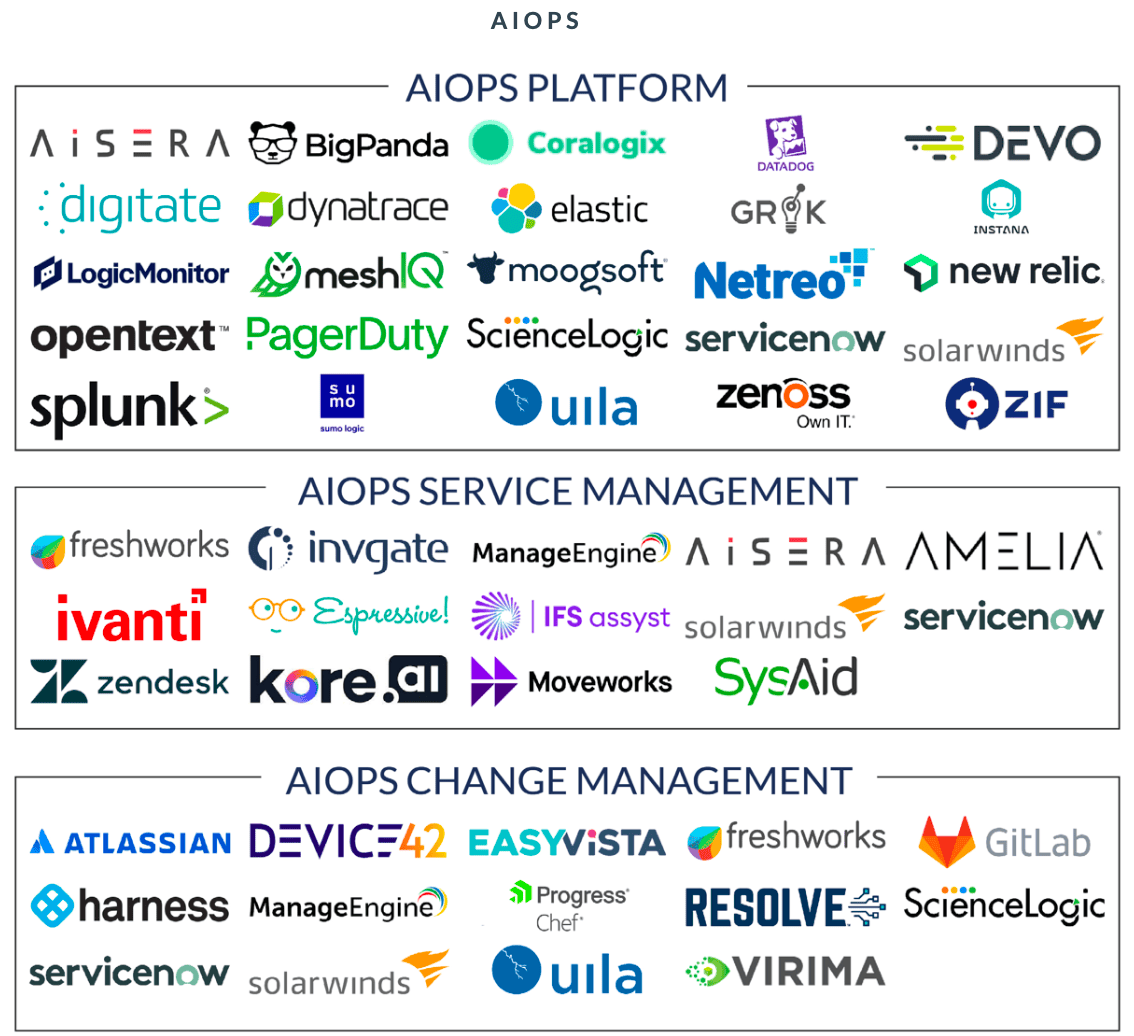
Enterprise Application - Conversational AI
Conversational AI has emerged as a transformative technological force in enterprise applications, revolutionizing how businesses interact with customers, streamline operations, and enhance productivity. At its core, conversational AI leverages advanced natural language processing (NLP) and machine learning algorithms to enable intelligent and contextually aware interactions between machines and humans.
In the enterprise context, these applications find diverse applications, from customer support chatbots and virtual assistants to internal process automation and data analysis tools. By harnessing the power of conversational AI, businesses can provide seamless, round-the-clock customer support, answer queries, resolve issues, and facilitate transactions in real time. This enhances customer satisfaction and significantly reduces operational costs by automating routine tasks, allowing human resources to focus on more complex and strategic aspects of business operations.
A distinguishing characteristic of conversational AI in enterprise applications lies in the ability to personalize interactions at scale. These applications can analyze vast and diverse datasets to understand customer and employee preferences, behaviors, and intents, enabling businesses to deliver tailored responses and recommendations. Through continuous learning, conversational AI systems become more adept at understanding nuanced language, context, and user emotions, creating a more natural and engaging conversational experience and improving the solution's effectiveness. Moreover, conversational AI finds extensive utility in internal corporate environments. It can streamline workflows, facilitate employee training, and automate data analysis, empowering organizations to operate more efficiently and make data-driven decisions.
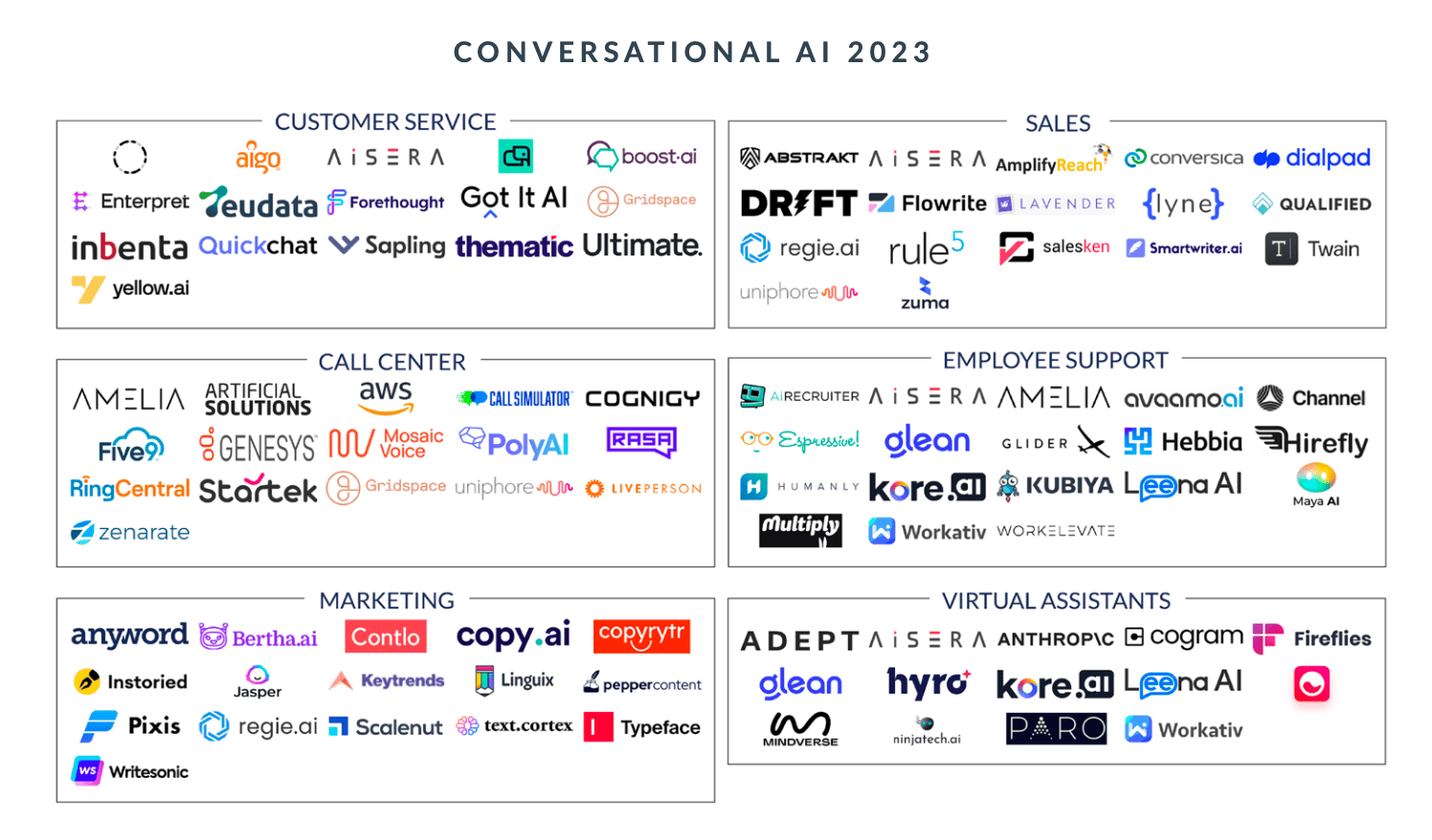
Business Practice Perspective
Technological solutions alone are insufficient; enterprises must adopt and cultivate aligned business practices that drive further value, efficiency, and effectiveness. These practices serve as the synergistic glue between the people, process, and technology of an organization (you can read more about the PPT framework here), strengthening the effectiveness of each in fulfilling their specific roles and functions within an organization.
The following represent some best practices for ensuring a thriving transition through the unfolding technological revolution.
Established Governance and Objectives
Implementing established governance and setting clear objectives are fundamental to successful AI integration. As a part of this, organizations must develop Key Performance Indicators (KPIs) and benchmarks to measure and improve AI effectiveness, reducing the risk of misuse, abuse, and harm. This also involves implementing collaborative cross-functional groups to further ensure and improve efficacy and organizational alignment. This practice fosters greater AI engagement, accountability, knowledge, and ROI across the business.
Targeted & Controlled Pilot Rollouts
Rather than large-scale implementations, utilize targeted and controlled pilot rollouts of AI and emerging technologies in specific, manageable areas. This approach allows businesses to assess real-world performance, identify challenges, and refine strategies before scaling up. Alongside targeted pilot rollouts, it’s also important to ensure adequate communication channels and feedback mechanisms are in place. Doing so leads to more effective application refinement and reinforces a culture of technological participation, learning, and innovation. These controlled and targeted rollouts are key in minimizing risk and enabling fine-tuning based on practical insights.
Employee Education & Training
AI tools only represent a part of the equation; these technologies will only be as effective as the individuals using them, resulting in the need for technically upskilled workforces. From this, it’s critically important to invest in the resources to educate and train employees on AI technologies, providing specific, tailored, and tangible use cases. Workforce understanding fosters greater adoption and encourages the integration of AI and other related technologies into daily operations and processes. Technical training programs, workshops, and resources enable the effective use of AI technologies, promoting a culture of innovation and ensuring that the workforce is equipped with the necessary skills and knowledge to utilize AI solutions.
Adaptability and Refinement
AI technologies continue to advance at an increasingly accelerated rate, requiring agile technological and operational posturing. To capitalize on current AI solutions while maintaining proactive adaptability to changing dynamics and landscapes, businesses need to take the following concurrent steps, which will leave them well poised to remain on the cutting edge of AI innovation:
- Identify and develop an enterprise-aligned solution
- Craft a strategic roadmap that takes into consideration the tech stack, capabilities, gaps, and future outcomes
- Implement focused, purposeful, and targeted pilot rollouts of AI technologies
- Maintain continuous feedback channels and mechanisms across functional groups
- Refine and adapt based on roadmap progression, objectives, and feedback
DevSecOps Frameworks
With any AI technology initiative, there must also be a prioritized integration of DevSecOps frameworks across the business. A culture and environment characterized by robust DevSecOps practices are important in maintaining customer trust, safeguarding sensitive data, and protecting against consequential cybersecurity vulnerabilities, attacks, and risks. This is particularly true in an environment with no regulatory clarity or certainty. DevSecOps ensures greater technological and operational effectiveness while providing secure and compliant-conscientious positioning. The current landscape of technological innovation, marked by the rapid advancement of generative AI technologies, is ushering in a new transformative era. We find ourselves in the early stages of an unfolding technological revolution, a period of irruption characterized by exponential growth and speculative investments. This irruption eventually leads to a frenzied phase, where technologies advance rapidly amidst uncertainty, instability, market turmoil, and regulatory pressures.
As this revolution unfolds, enterprises face a critical imperative to achieve improved competitive positioning and capitalize on the revolution: to develop robust strategies that align with the emerging paradigm. The deployment period, where optimization and consolidation occur, demands strategic technological investments and responsible management. Enterprises must embrace a multi-faceted approach, focusing on key technology areas, building technical capacity, and cultivating responsible technological governance practices to ensure alignment, effectiveness, and proactive posturing.
Moreover, in this era of rapid technological evolution, enterprises must not merely adopt technology but also cultivate corresponding business practices that reinforce that technology's value, efficiency, and effectiveness. Governance frameworks, targeted pilot rollouts, employee education, adaptability and refinement, and adherence to robust DevSecOps frameworks represent some of the critical strategies an enterprise must consider in adapting to the ongoing technological revolution.
Conclusion
The current landscape of technological innovation, marked by the rapid advancement of generative AI technologies, is ushering in a new transformative era. We find ourselves in the early stages of an unfolding technological revolution, a period of irruption characterized by exponential growth and speculative investments. This irruption eventually leads to a frenzied phase, where technologies advance rapidly amidst uncertainty, instability, market turmoil, and regulatory pressures.
As this revolution unfolds, enterprises face a critical imperative to achieve improved competitive positioning and capitalize on the revolution: to develop robust strategies that align with the emerging paradigm. The deployment period, where optimization and consolidation occur, demands strategic technological investments and responsible management. Enterprises must embrace a multi-faceted approach, focusing on key technology areas, building technical capacity, and cultivating responsible technological governance practices to ensure alignment, effectiveness, and proactive posturing.
Moreover, in this era of rapid technological evolution, enterprises must not merely adopt technology but also cultivate corresponding business practices that reinforce that technology's value, efficiency, and effectiveness. Governance frameworks, targeted pilot rollouts, employee education, adaptability and refinement, and adherence to robust DevSecOps frameworks represent some of the critical strategies an enterprise must consider in adapting to the ongoing technological revolution.
Navigating the evolving complexity of the innovation and technology landscape can be daunting. Vation Ventures can serve as your valued partner in your business journey through tailored market, technology, and competitive insights provided through Research as a Service or through our AI Readiness Assessment, a program designed to help CXOs transform operations, unlock innovation and gain a competitive edge through the understanding and use of AI. Get in touch today.