What is Data Intelligence?
Data is defining the 21st century. As a virtual commodity, it has infiltrated every form of business, from healthcare to manufacturing to retail and beyond. Data is extensive, and this big data is gathered, stored, and shared to make the wheels of business turn more smoothly. However, leveraging this data requires tools that can discover, analyze, and predict; the output from these tools is known as data intelligence. Using data analysis tools to spot trends and patterns, the information delivered using these tools allows a business to make predictions that help to inform better decisions and investment choices. We've teamed up with Arrow to help you better understand the landscape and leverage the data intelligence opportunities in front of you.
The path to accurate and effective data intelligence requires an effort that incorporates technology, processes, and people.
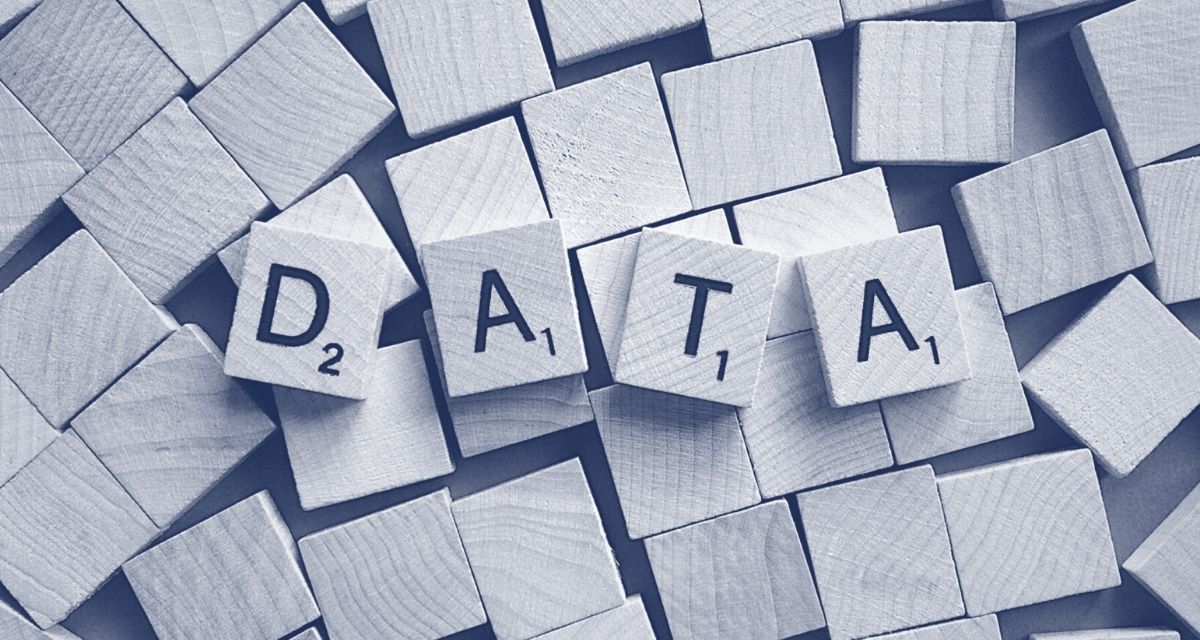
Why Is Data Intelligence Important?
Since the internet became ubiquitous and things became connected (IoT), data generation has increased exponentially. According to IBM, in 2016, humans had generated 90% of the world's data between 2014 and 2016 alone – this has only accelerated drastically in the past few years. In 2020, according to OpenVault, there was a 47% jump in data usage, driven by home working and other technology needed during the pandemic. All this generated data provides insights into consumer behavior patterns, potential fraud events, design options in product development, and many more important observations.
These insights can be harnessed using specialist tools that turn data into actionable items. Businesses can then use these actionable insights to make informed decisions on business operations, investments, product design, and development and improve customer relationships. An example is in the use of predictive analytics in marketing, used to inform a company about consumer behavior so that adjustments to marketing campaigns can be made.
What are the Technologies that Support Data Intelligence?
When the world started to increasingly gather, create, store, and share data, the development of technologies to manage this data emerged. In the area of data intelligence, this resulted in solutions that could discern all this data and understand often deeply hidden patterns and trends. The type of technology behind Data Intelligence is generally based on data analysis tools that use artificial intelligence (AI) and its subsets of machine learning (ML) and deep learning (DL).
Data Infrastructure
Data generated and created from online interactions, sensors, and all other data assets are the basis of data infrastructure. This data infrastructure comprises people, processes, and technology that interact with data assets. Open data increasingly forms a part of data infrastructure, moving away from a more siloed data posture. Data infrastructure aims to encompass all assets and actors within an ecosystem framework and secure and process data. Today, much of the data infrastructure is based in cloud systems and cloud data centers. Other vital parts of a data infrastructure ecosystem include open standards, data identifiers, and registers of data.
Data Analytics
All this data is nothing without an understanding of the data itself. This intelligence is formed using data analytics, which offers insights into what the data represents. Data analysis interprets gathered data using logical reasoning and is typically based on machine learning/deep learning algorithms. TIBCO and Tableau both provide excellent data analytics tools. TIBCO gives organizations easy-to-use dashboards so anyone in the organization can benefit from predictive and streaming analytics. Tableau integrates with CRM platforms and other data sources to provide in-depth data analytics and visualization of enterprise interactions.
Data Governance
Data governance combines the processes and procedures needed to deliver best practice data asset management. Data governance provides internal data standards and policies designed to manage data usability, availably, security, and integrity. Good data governance policies ensure that data is standardized and of high quality to provide accurate insights and business intelligence.
Artificial Intelligence/Machine Learning
The technologies behind extensive data analysis for data intelligence are typically based on artificial intelligence (AI) and its subset of machine learning (ML). These methodologies have been around for decades but only recently been harnessed for use with big data. AI is a term used to describe computational functionality that mimics human learning. AI is used to automate decisions made by machines; in doing so, repetitive tasks can be automated.
ML algorithms are trained using large data sets; the more data provided for training, the more accurate the system becomes at pattern recognition. There are several types of ML models, but the two most frequently mentioned are 'supervised' and 'unsupervised' learning. The model that's best for a given task depends on the use case.
Enterprise Applications
Data is transforming the enterprise and the business units within it. For example, in sales and marketing, data across various sources, from marketing campaigns, previous sales, customer engagement programs, social media, and so on, can be collated and analyzed to provide fuller insights. More accurate customer profiles can be developed using big data analyzed using ML. In other areas such as logistics, data intelligence and AI can analyze a vast number of variables to plan for events and create accurate delivery schedules, etc. Across the enterprise, data intelligence is helping departments to reduce costs and improve operations.
Industry Applications
Data offers actionable insights that benefit many industries. Some examples of big data and AI applications across industries include:
- Manufacturing: AI in manufacturing is driving the Smart Factory 4.0. Extensive data analysis is being used across all aspects of the manufacturing lifecycle, from product design to the manufacturing process to maintenance to marketing and sales to logistics and delivery. Sisense focuses on connecting operations in manufacturing with IoT device data in one centralized platform.
- Financial: ML is used in various situations, including monitoring financial market activity and anti-fraud measures.
- Healthcare: ML and other types of AI are being used in healthcare for a variety of applications, including personalized care and improving patient outcomes.
Other industries using AI and data intelligence include government, insurance, utilities, and education.
Data Sources
Data sources are varied, and the choice of a data source is dependent on the requirement. A data source can be anything where data is created, generated, or gathered (aggregated). There are primary and secondary data sources that can be in the form of structured data, unstructured data, and semi-structured data.
Typical data sources include
- Web services
- A database
- Spreadsheets
- Sensors, e.g., via an IoT device
- ERP (Enterprise Resource Planning)
- CRM (Customer Relationship Management) systems
Data sources typically use secure protocols to communicate data and connect to other systems that will consume this data via an Application Programming Interface (API). Data from sources can be historical or real-time.
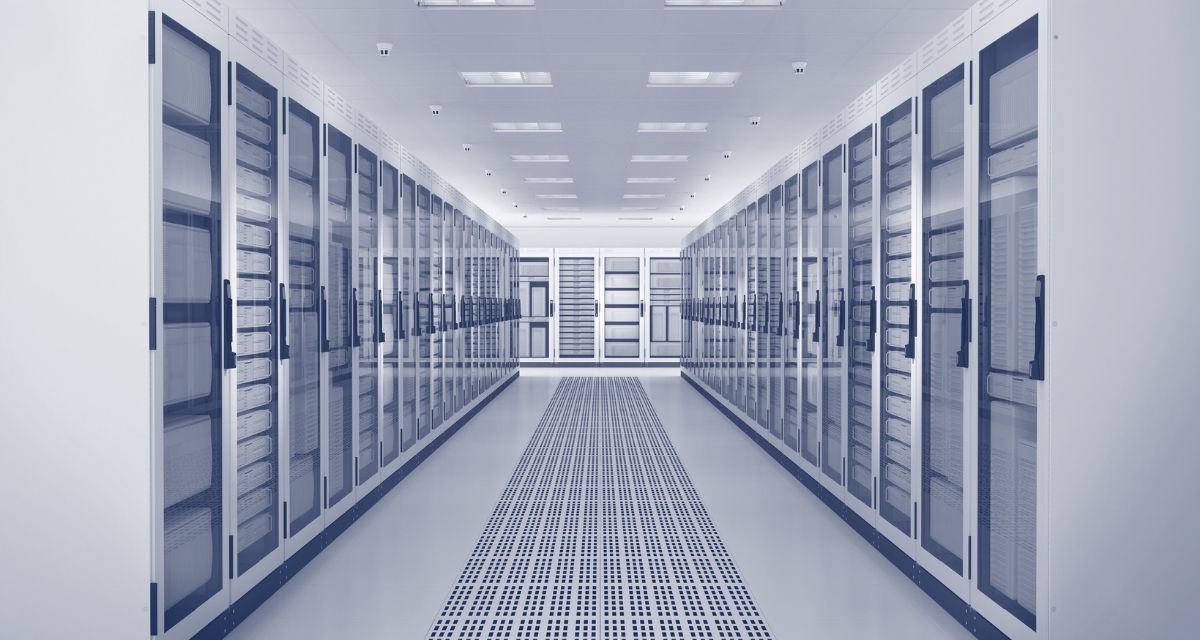
Data Intelligence Technology Trends and Disruptions
Data intelligence is where technology and business strategy dovetail; it is an exciting area of technology that uses innovative technologies such as artificial intelligence (AI). The disruptive force behind big data is facilitated using tools provided by a large ecosystem of Data Intelligence vendors.
Modern Data Stack (MDS)
The Modern Data Stack (MDS) is a new data integration model based in the cloud. An MDS is not typically delivered by a single tool but rather a series of integrated components covering the entire data lifecycle. The goal of MDS is to analyze business data while improving efficiencies.
Data Ingestion: Data loading can be a complex job, especially where there are multiple data sources and types. Data Ingestion companies are disrupting the space by automating data ingestion. Fivetran and Matillion are disrupting the space by providing exceptional data ingestion capabilities as part of an ELT (Extract, Load, Transform) solution. For example, both Fivetran and Matillion can connect to multiple data sources and run data transformations to prepare data for analysis during the extraction step in ELT.
Data Warehouse: Data warehouses are used to aggregate data across the business and store data in a relational database. This data can then be organized and analyzed. In the past, these warehouses were expensive and limited in the types of analysis supported. Subsequent generation data warehousing is much faster and cost-effective. Modern data warehouses typically use ELT platforms to prepare and transform data for ingestion. Two disrupter companies working in data warehousing are Snowflake and Yellowbrick; the two companies provide the L in ELT. Snowflake is a cloud-native data warehouse service that is an extension of Amazon Web Services or Microsoft Azure cloud infrastructure. No hardware or software installs are required. Yellowbrick uses a distributed cloud infrastructure to deliver data warehouses across a hybrid cloud model.
Data Transformation: Data comes in many forms across several structures. Data transformation converts data from one format or structure to another. This process is a fundamental step in data intelligence and ELT, converting the data to a standard that can be used for data analysis. Preparing and migrating data before transformation can be complex and time-consuming; it can also be prone to errors. Two companies disrupting the data transformation space are Dataform and DBT (Data Building Tool); the companies provide the T in ELT. Dataform is GUI driven and based on BigQuery (a cloud-based data warehouse). Dataform enables data analysts to take raw data and turn it into clean data sets ready for analysis. DBT is a dedicated tool that supports analysts by enabling them to write a "select" script to transform data, e.g., which data field to select from a database.
Business Intelligence (BI): BI uses a mix of technologies, architectures, and processes to analyze business information for actionable insights. BI focuses on business operations and how to optimize business processes and make better business decisions, e.g., how to cut costs, make a business process more efficient, etc. BI uses data analysis, reporting, performance management, and information delivery to inform business decisions. In this way, BI and DI are intrinsically connected. Business intelligence, however, places focus on enhancing and optimizing business operations in the present, i.e., how to run a better business; data intelligence places focus is on using data for prediction. The rise of importance in customer-centric business models and big data applied to business strategy is helping to disrupt the applications of BI. Sisense and Thoughtspot are two companies that are working in this space. Sisense offers a way to quickly analyze data without the need to call in the IT department. This makes Sisense accessible to companies of all sizes. Thoughtspot is another easy-to-use BI offering. It is a highly visual platform, offering dashboards to analyze data and create reports with no IT help.
Machine Learning Operations (MLOps)
MLOps is a culture and practice where data engineering (ML) and operation staff (Ops) work together to manage the deployment into the production of machine learning systems. MLOps combines the resources needed to train and deploy into continuous production ML algorithms within a business context.
Data Preparation: Data needs to be prepared for ML analysis and transformed into a form used by the ML system. Preparation involves a data split, which creates data for training and testing that can be used to evaluate an ML model. Data preparation is an intensive part of the data intelligence process. Reducing the burden of this step using automated techniques has enabled disrupter companies to enter the space. Two such companies are Paxata and Trifacta. Paxata, recently acquired by DataRobot, pioneered the development of self-service data preparation, making it easier and more controllable for companies to develop an MLOps culture. Trifacta provides a web interface to upload data and prepare it for use in ML models.
Machine Learning Model Development (AutoML) Designing and building ML models for the process is one of the most challenging tasks of data intelligence. One of the issues being too many ML model choices. Companies that offer an automated ML development platform (AutoML) are making headway in this complicated area. Two companies that are disruptive in this space are Dataiku and DataRobot. Both Dataiku and DataRobot provide a visual AutoML interface that can use data in spreadsheet form as a basis for ML model choice. DataRobot requires no coding skills to run the ML optimization process. Dataiku has more flexibility and allows for some design input.
Machine Learning Model Deployment Once an ML model has been chosen, it needs to be deployed into production. This involves a process that is dependent on the scenario and the context of the deployment. There are several types of ML model deployment:
- One-off (local): Performed ad-hoc on a local machine; often used to prototype ML models.
- Batch: Performed by running predictions regularly, e.g., what is next month's revenue likely to be. AutoML is often used with batch training as it automates the choice of model.
- Real-time: Running an ML model on-demand to make predictions as needed. Online machine learning models support this method.
The Transform 2019 conference highlighted that 87% of data science projects never make it into production. This has created a gap for disrupters such as Algorithmia and Seldon to enter. Algorithmia offers an MLOps platform that manages the entire lifecycle of ML models into production. Seldon uses Kubernetes to deploy ML projects at scale.
Machine Learning Model Management (MLMM): MLMM is an integral piece of the MLOps pipeline. Machine Learning Model Management is a policy-based approach that incorporates all aspects of the ML model lifecycle, including creation/choice, training, versioning, and deployment. Model management also incorporates regulations and compliance, tracking and audit of ML versions and metrics, and packaging of models for reproducibility in deployment. Domino Data Labs and Fiddler Labs are disrupters in this sector of the data intelligence industry. Domino Data Labs offers unified analytics via a Platforms-as-a-Service (PaaS) to speed up the development and deployment of ML projects and automate versioning. Fiddler Labs provide 'Explainable AI' to accelerate the MLOps process. In doing so, Fiddler delivers a way to bridge the gap between the skills of data scientists and the business teams applying actionable data intelligence.
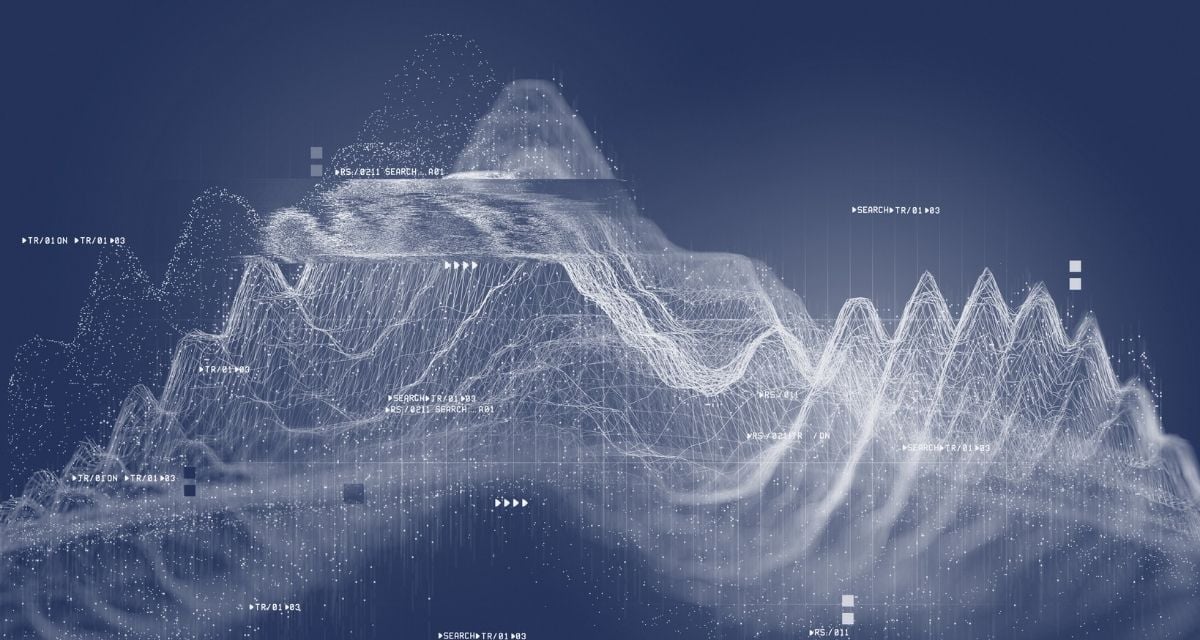
CXO Priorities within Data Intelligence
The C-Suite is setting priorities based on what data intelligence can offer and the culture of customer-centric business models. These priorities include:
Self-Service Data
Data scientists are in demand because of the increasing use of data intelligence practices. However, there is a skills gap, with a shortfall of 250,000 data science professionals. A Self-Service Data model is offered by specialist ELT tools that manage data to deliver ML projects to production, even when a company has no data science experts in-house.
Data-In-Place
Data-In-Place or "In-Situ" machine learning, developed by Dr. Changran Liu of TigerGraph, is a new way to perform machine learning. Traditional ML models require that data is extracted from a database and used to train an ML model, which is then used to enrich the database. In-Situ or Data-In-Place models allow ML algorithms to utilize the data without extraction. Benefits include cost savings, prevention of data leaks, and the advantage of using fast-changing data sets with continuous ML model evolution.
Artificial Intelligence/Machine Learning
Artificial intelligence (AI) and machine learning (ML) are the technologies behind data intelligence. These technologies can analyze big data and deliver insights, which are then used to create better customer relationships and experiences in a customer-centric, omnichannel world. Today, many repetitive tasks are automated using AI algorithms. ML uses data to learn. The more data fed into an ML algorithm, the better the algorithm performs a task.
Data Monetization
Many companies now use Data Monetization as a revenue stream. A study from McKinsey, into data and analytics shows that increasingly, companies are using Data Monetization to generate growth. According to the study, Data Monetization enables a company to use data to develop new business models, products and enhance existing offerings. Data Monetization requires that a company provides access to held data to third parties. To optimize Data Monetization, a company should develop a Data Monetization Strategy. KPMG sum this up as: "Data monetization is about effective and timely exploitation of an ever-growing new class of asset, the enterprise data, and converting that asset into currency (profits) by increasing revenues or decreasing costs."
What is the Channel Impact on Data Intelligence?
As data becomes more pervasive and the stack continues to evolve, end customers will increasingly turn to the channel for consultative guidance to deploy these technologies. The sheer volume of new market entrants in the Modern Data Stack and Machine Learning Operations areas will create opportunities for solution providers to orchestrate the ecosystem on behalf of their clients to create the desired business outcomes.
Distributors like Arrow are in a great position to help solution providers pull it all together with platforms like ArrowSphere. ArrowSphere enables the solution provider to easily and efficiently manage operations for their cloud vendors through a single interface that handles quoting, provisioning, billing, governance and analytics.
In terms of their overall approach, solution providers will need to adjust and add capabilities in the following areas:
Sales: Account managers will need to expand their target personas to capture the increasing data intelligence opportunity. New targets include external business unit owners (e.g., marketing), business analysts, and data engineers. They will also be well served, targeting data-intensive vertical markets such as Banking & Finance, Healthcare, and Retail.
Pre-sales: Data intelligence is still a specialized and consultative sale requiring the solution provider to have subject matter expertise on staff. Solution providers should consider dedicated specialty sales resources, engineers versed in data architecture and design, and solid proof of concept capability.
Practice Development: Because data iIntelligence is foundationally a process-driven solution encompassing people, processes, and tools, solution providers should consider building a DI practice to define its consulting methodology, manage its vendor alliance portfolio and ensure its people are trained and certified.
Staying on top of new technologies is a constant battle for organizations - we can help.
You're focused on your business in the same way we're focused on innovation and trends in technology because that is our business. We offset the immense time, research, and costs you spend on identifying technologies to solve your organization's problems. Interested in learning more? Let's work together.